Keywords
|
acoustic sensor; hyperbolic circular array; acoustic soure, localizations |
INTRODUCTION
|
Sound Source Localization (SSL) using Sensor Network application at forest is one of the emerging research topics since the early 1990’s. Acoustic Circular Array is fixed for while covering the specific target in outdoor environment. In our real time implementation the outdoor environment (forests) can be extremely affected by external factors[1]-[3]. Elephant sounds are recorded in sensor board at the same time other sounds emitted by other source are also recorded. We have fixed 8 sensor for our application to estimate direction at forest and 4 Sensors circularly covered around (maximum) 3 kms for estimating the Direction of Arrival and remaining sensors used for recording the Temperature, Wind Speed, Wind direction and Humidity. Sensor positions are arranged as (Top,Bottom,Left,Right or East/West/North/South). Database Management System was processed for Sound recorded at different locations. The file name stored in sensor’s are related to their original direction(East,west,north and south)[4][6]. Due to limited battery power efficiency sensor’s are enable mode if they sense the signal otherwise all the nodes are sleep mode. As soon as sensor detects the signal, acoustic fingerprint(Database)is designed and signal pre processing (A/D conversion, Filtering and Compression) are done. Using the pre processed signal SPIN routing protocol is designed. The purpose of using SPIN protocol is to eliminate redundancy. |
In our real time experimental analysis we have faced the following problem and rectified [7]. If two sensor’s store a similar signal in exactly the same time. SPIN routing protocol is designed to eliminate the signal redundancy occurred in sensor. In order to use the battery energy efficient the sensor network operation used here is synchronous protocol[8]-[10]. After estimating the DOA arrival we are identified and work some of the few external factors affecting outdoor propagation in forest. The external factors are (i) temperature changing the speed of sound profile (iii) wind speed and direction changing the speed of sound profile iii)reverberation inside the forest(echo,scattering,relection) iv)diffraction by barriers and other considerations. |
The rest of the paper is structured as follows. Chapter 2 discusses the existing approaches for finding the Direction of Arrival. Chapter 3 explains the proposed methodology for DOA estimation. Chapter 4 reveals the experimental results for DOA estimation and Error Analysis for external factor affecting outdoor propagation in forest. Chapter 5 outlines the Results and Discussion. Finally, Chapter 6 concludes the paper. |
REVIEW OF EXISTING APPROACHES
|
Specific target DOA estimation in forest is one of the essential sensor network applications in forest. Estimating DOA for an acoustic source of narrow band sources where origin of the source is far away from the sensor array optimal algorithm are processed in existing techniques such as multiple signal classification (MUSIC) the minimum variance method of Capon , estimation of signal parameters via rotational invariance technique (ESPRIT) and more. Better performance was achieved at higher the SNR[10]-14]. The standard method for estimating DOA is ML method. |
Observed parameters are essential for formulating likelihood function. Estimation of ML with respect to all unknown parameters, which may include the source DOA angles, the signal covariance, and the noise parameters by maximizing the likelihood function.There are different optimization techniques available in literature for optimization of ML function like AP-AML, simulated annealing (SA) , genetic algorithms (GA) fast EM and SAGE algorithms and a local search technique e.g. Quasi-Newton methods[13]. The evolutionary algorithms like genetic algorithm , particle swarm optimization and simulated annealing [can be designed to optimize the ML function. Genetic algorithm and particle swarm optimization had already used as a global optimization technique to estimate the DOA for uniform array[15]. |
PROPOSED METHODOLOGY AND DATA FOR RESEARCH
|
Problem Identification |
Let us consider an circular omni-directional array of n sensor which sense the random signal generated at least one target coverage etc, from the specific target around 3 km’s with the unknown location or Direction of Arrival (DOA), w be the direction of sensor located in the forest, L be the life time of a sensor, dc be the set of directions that cover a target[6][18]. |
Signal Generation in Hyperbolic Circular array |
The prototype for all Hyperbolic Partial Differential equations of one-way wave equations |
ut +aux= 0 (1) |
where a is a constant, t represents time, and x represents the spatial variable. |
Before estimating the Direction of Arrival, the recorded signals are pre processed. After pre processing the digital signal is stored in the Acoustic Fingerprint. Routing Protocols are designed for data transmission between acoustic sensor network. Due to limited battery power efficiency the sensor network are divided in to three modules[13]. |
(i) Sensor identification and Management |
(ii) Routing Protocol |
(iii) Network Topology |
The following figure represents the signal pre processing results before direction of arrival estimation. |
EXPERIMENTAL RESULTS AND DISCUSSIONS
|
Due to several external factors our DOA accuracy is affected in densed forest. Due to heavy wind speed , the elephant emits the sound far away from the sensor the results are affected by various metrological condition such as(wind speed and temperature) etc. The following figure shows the doa estimation for an stand alone elephant in the palamalai hill. Due to heavy wind speed DOA results are highly affected by error rate. |
Factors Affecting Outdoor Sound Propagation In Forest |
In our real time implemention using acoustic sensor at forest we are facing the following external factors affecting the sound propagation at forest[16]-18]. They are listed as follows, |
1. Attenuation from geometrical divergence Distance between source and sensor (near & far away) |
2. Wind changing the speed of sound profile |
3. Temperature changing the speed of sound profile |
4. Reverberation inside the forest(Echo) |
5. Types of source-Point, Enlarge and Medium objects |
6. Humidity affecting the speed of sound profile |
7. Diffraction by Barriers (trees and vegetation) and other considerations(reflection,scattering0 etc., |
DATA FOR RESEARCH |
The following figure show the acoustic data collected from mudumalai hills. The parameters for elephant sound recordings are listed as follows. |
TARGET CATEGORY : 1)ESCALATION -WALKING |
TOTAL NO. OF TARGETS : 3 |
WIND SPEED : 5 miles/hr |
WIND DIR : EAST |
TEMP : MAX-72 F, MIN-22 C |
RELATIVE HUMIDITY : 74% |
HEIGHT ABOVE GROUND : 12fT |
RECORDING SOUND TIME AND DATE : 10 AM , 10/12/2012 |
RECORDING LOCATION : MUDUMALAI FOREST |
LATITUDE :11.57 |
LONGITUDE : 76.59 |
SAMPLING FREQUENCY : 500 micro sec |
ELEPHANT TYPE : BABY/ADOLESCENT/ ADULT HOOD |
FILE NAME : *3.WAV |
VISIBILITY : 10KM |
CLOUD COVERAGE : 5% |
RESULTS AND DISCUSSIONS
|
Our proposed results show that due to some external factors the direction of arrival estimation or source localization error are affected. Due to several external factors at forest the source localization is affected by the following reasons. (i) humidity and temperature (ii) enlarged object bear by sensor (iii) reverberation inside the forest(echo). Due to diffraction by barriers,trees and vegetation when the object emit the sound when the wind speed is about 5 miles/hour with in sensor and source with in nearby (25ft) sensor field the source localization achieves high accuracy. Otherwise source and sensor far away from sensor field, temperature variation and reverberation in forest (echo) affects the localization error. |
CONCLUSION AND FUTURE SCOPE
|
This paper, we proposed a methodology for estimating direction of arrival and error analysis for outdoor atmospheric conditions using hyperbolic partial circular array. Our DOA estimation results are very encouraging about using limited battery power and minimum number of sensor nodes. In future our work enhances in two directions (i) identifying more external factors (type of ground surface, thunder, lightning, under water) affecting direction of arrival. (ii) Simultaneously monitor and estimating the direction of arrival for more than one source. |
Figures at a glance
|
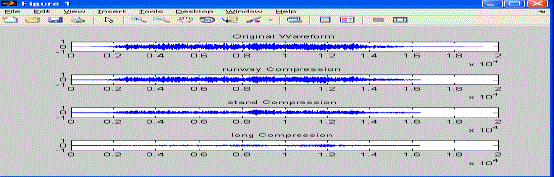 |
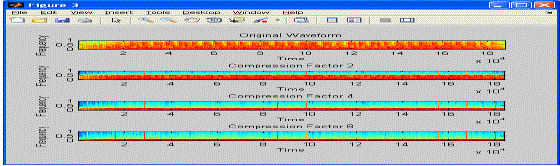 |
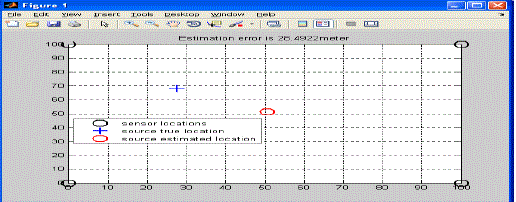 |
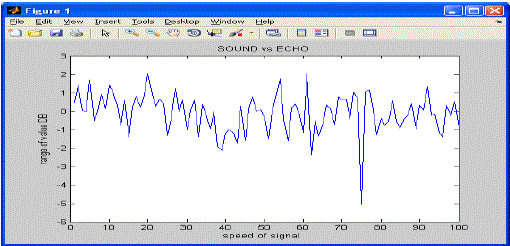 |
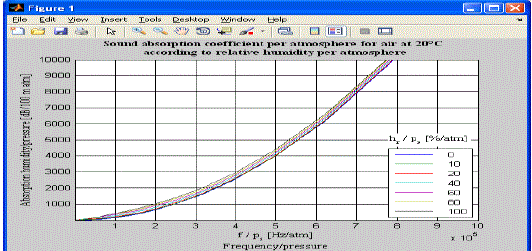 |
Figure 1 |
Figure 2 |
Figure 3 |
Figure 4 |
Figure 5 |
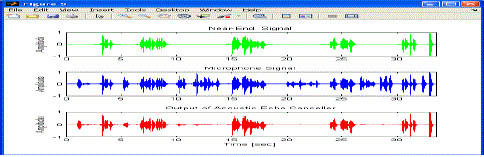 |
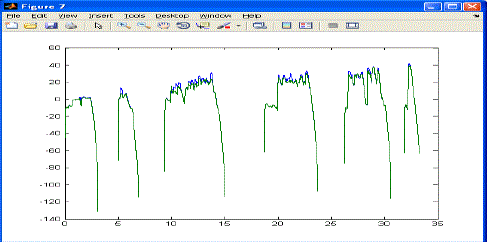 |
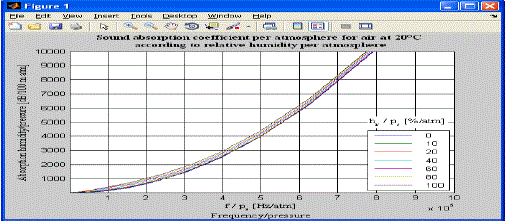 |
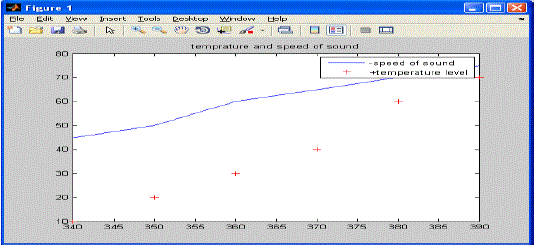 |
Figure 6 |
Figure 7 |
Figure 8 |
Figure 9 |
|
References
|
- J. Haitsma and T. Kalker, “A Highly Robust Audio Fingerprinting System,” in Proceedings of the International Symposium on MusicInformation Retrieval, Paris, France, 2002.. (2004).pp 1-2
- Cano, P., E. Batlle, T. Kalker, and J. Haitsma.. A review of audio fingerprinting. The Journal of VLSI Signal Processing pp.2-3
- Haitsma, J., T. Kalker, and J. Oostveen. Robust audio hashing for content identification. Proc. Of the Content-Based Multimedia Indexing pp3-4.
- S.Poornachandra, B.Sasikala Digital Signal ProcessingDigital Signal Processing, Vijay Nicole imprints Private Limited. ISBN: 81-8209-.pp 4-5.
- M. Brandstein and D. Ward, Eds., Microphone Arrays: Signal Processing Techniques and Applications, Springer, 2001. Pp 4-6
- B.Somanathan Nair., Digital Signal Processing Theory, Analysis and digital Filter Design(textbook) prentice hall of india private limited.
- D.B.Ward and R.C.Williamson,“Particle filter beamforming for acoustic source localization in a reverberant environment,”in Proc. IEEE ICASSP, Orlando, FL, USA, May 2002.
- J. Haitsma, T. Kalker, and J. Oostveen. An efficient database search strategy for audio fingerprinting. In IEEE workshop on Multimedia Signal Processing, St. Thomas, USVI, 2002.
- M. Stevenson, V. Stanford, 3 and C. Rochet3 An Automated Acoustic SystemtoMonitor and Classify Birds Hindawi PublishingCorporation EURASIP Journal on Applied Signal Processing Volume 2006, Article ID 96706, Pages 1–19
- T. Gustafsson, B. Rao, and M. Trived “Source localization in rever-berant environments: performance bounds and m estimation,” inProc.of ICASSP, 2001.
- Y. S. Huang, Y. P. Huang, K. N. Huang, and M. S. Young “An accurate air temperature measurement system based on an envelope pulsed ultrasonic time-of-flight technique”, Rev. Sci. Instrum. 78, 115102, 2007.
- S. M. Kay, Fundamentals of Statistical Signal Processing:Estimation Theory. Englewood Cliffs, NJ: Prentice-Hall, vol. I, 1993.
- N. Patwari, A. O. Hero , M. Perkins, N. S. Correal, “Relative Location Estimation in Wireless Sensor Networks”, IEEE transactions on signal processing, vol. 51, No. 8, Aug 2003.
- K. Delin, S. Jackson, D. Johnson, S. Burleigh, R.Woodrow, J. McAuley, J. Dohm, F. Ip, T. Ferre, D. Rucker, and V. Baker, “Environmental studies with the sensor web: Principles and practice,” Sensors 5, pp. 103–117, 2005.
- D. Culler, D. Estrin, and M. Srivastava, “Overview of sensor networks,” IEEE Computer 37, pp. 41–49, Aug. 2004.
- Chinthaka M. Dissanayake Propagation Constraints in Elephant Localization Using an Acoustic Sensor Network 978-1-4673-1975
- J. V. Wijayakulasooriya, “Automatic Recognition of Elephant Infrasound Calls Using Formant Analysis and Hidden Markov Model”, 6thInternational Conference on Industrial and Information Systems, Sri Lanka, Aug. 2011.
- W. R. Langbauer, K. B. Payne, R. A. Charif, L. Rapaport, F. Osborn, “African elephants respond to distant playbacks of low-frequency conspecific calls”, J. Exp. Biol. 157: 35-46, 1991.
|