INTRODUCTION
|
Distributed denial-of-service (DDoS) bouts pose an immense danger to the Internet, and many defense deviceshave been proposed to battle the problem. Attackers con stantlyadjust their tools to avoid these security systems,and researchers in go modify their approaches to grip new attacks. The DDoS field is rapidly becoming more andmore multifaceted, and has reached the point where it is problematic to see the forest for the plants. On one hand, this delaysan understanding of the DDoSmarvel. The variety ofrecognized attacks creates the stamp that the problem spaceis vast, and hard to travel and address. On the other hand,current defense systems organize various plans to counter the problem, and it is problematic to comprehend their resemblances and changes, measure their efficiency and cost, andto liken them to each extra.This paper proposes a classification of DDoS attacks and aclassification of DDoS defense systems. Composed, they structure the DDoSarena and facilitate a worldwide view of the problem and answer space. By setting apart and highlightingcrucial features of attack and protection mechanisms, while abstracting detailed changes, these classifications can be usedby researchers to response many important questions:What are the different ways of committing a DDoS attack? Why is DDoS a problematic problem to handle?Whatbouts have been handled efficiently by existing defense schemes? What attacks still continue unaddressed and why?Given two protection mechanisms, A and B, how wouldthey perform if bout C occurred? What are theirsusceptibilities? Can they complement each additional andhow? Are there someplacement points that are better right for A than B and vice versa?² How can I donate to the DDoSfield?The proposed classifications are complete in the followingintelligence: the attack taxonomy covers known bouts and alsothose which have not hitherto appeared but are truthful potential threats that would touch current defense devices;the defense system classification covers not only published methods but also some commercial methods that are sufficiently documented to beexamined. Along with classification, we provide illustrative examples of existing mechanisms. We do not right that these classifications are as detailed aslikely. Many classes could be alienated into several deeperheights. Also, new attack and protection mechanisms are likelyto seem, thus adding new lessons to the ones we propose.Our goalmouth was to select several significant features of attackand defense devices that might help researchers designgroundbreaking solutions, and to use these topographies as classification criteria. It was also significant not to confuse thebooklover with a too intricate and detailed organization. It isour hope that our work will be additional extended by additionalresearchers.We also do not claim that classes gulf attacks and defenses in anhigh-class manner, i.e. that an example of anattack or a specific defense system must be secret intoa single class based on a given standard. It is possible foranbout or defense to be comprised of numerous mechanisms, each of them fitting to a different class.The complexity and width of the proposed classifications are notsuitable for a old-style numbering of headings { numbers would rapidly become too elaborate to follow. We thereforepresent a customized marking (numbering) of subsetheadings in Sections 3 and 5. Each organization criterionis marked shortening its name. Attack classes below thiscriterion are marked by the standardcontraction and an number, connected by a sprint. To indicate depth ofaexact criterion or a class in the classification, the completemark of a subset is generated by traversing the classifications depicted in Figure 1 and Figure 2, from root to thething in question, concatenating heights with a colon. |
LITERETURE SURVEY
|
A denial-of-service attack is branded by an explicitattempt to prevent the genuine use of a service [14]. Adispersed denial-of-service attack organizes multiple attacking entities to reach this goal. This paper is exclusively concerned with DDoSbouts in the computer kingdom, perpetrated by causing the prey to receive malicious circulation and suffer some damage as aimportance.One frequently exercised way to perform a DDoS attack is for the assailant to send a stream of packs to avictim; this stream eats some key resource, thus version it unavailable to the prey's legitimate clients. Additional common approach is for the assailant to send a fewmalformed packs that confuse an application or a procedure on the victim mechanism and force it to freeze or restart.In September 2002 there was anstart of attacks that loaded the Internet infrastructure rather than directing specific victims [5]. Yet another likely way to deny serviceis to undermine machines in a prey network and consumesome key reserve so that legitimate customers from the samenetwork cannot get some inside or outside facility. Thislist is far from thorough. It is certain that there are numerousother ways to deny facility on the Internet, certain of whichwe cannot forecast, and these will only be exposed afterthey have been browbeaten in a large attack.What makes DDoSbouts possible? Current Internet design emphases on effectiveness in touching packets fromthe source to the destination. This project follows the end-to-end example: the intermediate network delivers the bare minimum, best exertion packet forwarding service, sendoff tothe sender and the headset the deployment of advanced procedures to achieve desired service assurances such as qualityof facility, reliable and robust conveyance or security. Theend-to-end example pushes the complexity to end crowds,leaving the intermediate network humble and optimized for pack forwarding. There is one unlucky implication.If one party in twoway message (sender or receiver)disobeys, it can do arbitrary injury to its peer. No onein the middle network will step in and stop it,sinceInternet is not intended to police traffic. One importanceof this policy is the attendance of IP spoofing 1. AdditionalareDDoS attacks. The Internet project raises several securitysubjects concerning chances for DDoSattacks.Internet security is extremely interdependent. DDoSattacksareusually launched from schemes that are subvertedfinished security-related negotiations. Regardless of howwell tenable the prey system may be, its vulnerabilitytoDDoS attacks be contingent on the state of safety in the rest ofthe worldwide Internet [21].Internet capitals are limited. Each Internet object (host,network, service) has incomplete resources that can be spent by too many users.Intellect and resources are not collocated. An end-to-end message paradigm led to storing greatest of the intelligence wanted for service assurances with end hosts, warning the amount of dispensation in the intermediate networkso that packs could be forwarded rapidly and at minimalcost. At the similar time, a desire for large amount led tothe design of high bandwidth trails in the middlenetwork, while the end networks capitalized in only as muchbandwidth as they supposed they might need. Thus, hateful clients can misuse the plentiful resources of the unknowing intermediate network for distribution of numerous messagesto a less provisioned prey.Accountability is not compulsory. IP spoofing gives assailantsa powerful mechanism to seepage accountability for their movements, and sometimes even the incomes to perpetrate attacks(re°ector attacks2 [59], such as the Smurf bout [10]). |
SYSTEM ARCHITECTURE
|
In statistical-based method it concludes normal network act and then all circulation that deviates from the usual is marked as anomalous. This method is used to learn network circulation prototype on a specific network. By examine network circulation and processing the information with multifaceted statistical procedures, this systems look for irregularities in the established normal network circulation patterns. All packs are given an irregularity score and if the irregularity score is higher than a sure threshold, the intrusion credit system will generate an alert. |
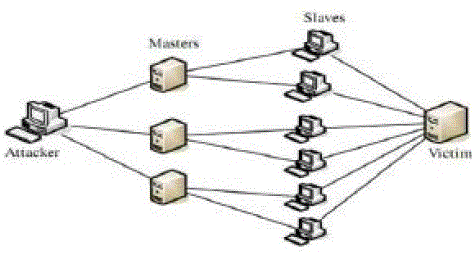 |
This method has a number of compensations. It is capable of detecting new hidden attacks like denial of facilities attacks, worm or worm. It is also capable of noticing low intensity slow step attacks. Another major advantage of this approach is that it is possibly easier to maintain than a law based approach since we do not need to uphold and update any best of signature. The basic problematic with this type of method is the selection of appropriate verge value. Problem of false optimistic and false bad occurs due to this worth. If value is set low than relation of false positive upsurge if value is set too tall than the anomalous doings cannot be verify means untrue negative increases. |
IMPLEMENTATION
|
A. Anomaly Detection
|
The two mostly common techniques to noticing web-based attacks are signature-based discovery and anomaly based discovery. Signature-based detection trusts on detecting patterns of recognized attacks to recognize hateful behavior. While they are precise, they have to be kept up-to-date with present attacks to be lively. Any bouts that are not in the signature or design database will therefore not be noticed. This weakness can be burdened by creating diverse forms of a single attack. Anomaly-based discovery relies on statistical examination of the data to and presentation that deviates from the usual activity. One of the big compensations over signature based bouts, if used correctly, is that it is talented to detect difference of bouts or even totally new bouts. However, this could also consequence in normal movement existence standard as malicious. |
B. Anomaly Discovery of Web-based Attacks:
|
The irregularity detection clarify by Kruegel and Vigna[19] works on personality needs. The center is mainly on the detection of varied data input related bouts, by analyzing various features of the request path of allrequest. The URI linked with each request (minus the domain name) is unglued into three parts. The trail, which consists of the resource path and program, and the curb and their values. A program in this setting, also called a reserve, is dined by the last share of the path in the URI beforehand the parameters start. Only HTTP GET needs that generated a reply code by the web waiter indicating success1 were used. This dataset is additional reduced by removing any requests that do not cover any query limits. |
C. Spectrogram:
|
Song et al.[20] describe a scheme, which is parallel to examine separate HTTP requests, but operating on a inferior level. The major difference is that together HTTP GET and HTTP POST supplies are examine and the whole request path including inquiry parameters are treated as a solitary object. For a POST request, the appeal body containing the POST data is too used. It uses a group of n-grams and Markov manacles to calculate an irregularity score for this specific request. The given cord is scanned and probabilities are careful for the succession of characters that happen in this string. It usages anticipation Maximization to and the best settings given the gram-size and the amount of Markov chains to use in the exercise phase. The Spectrogram scheme was tested using actual data from two university web servers which was calm over a period of a month. These waiters contained various writings for the computer science section and personal homepages of scholars. Both of which can be stimulating targets for attackers. Standardization is performed on the calm data by un-escaping cords, removing whitespaces and statistics and converting all fonts to lowercase. A manual review of the data ensures that the dataset does not cover attacks of any caring. Finally all identical requests are removed to stop creating a bias towards needs that occur more frequently than others. The subsequent dataset was then used to train the perfect. The attack-data includes distant le inclusion attacks, JavaScript and XSS, attacks, SQL injection and many sole shell code examples. The results were general pretty good, with excellent results in detecting larvae, shell code attacks, SQL and XSS attacks. |
D. Detecting Anomalous and Unknown Interruption against Pro-grams:
|
Employing a neural network to notice malicious activity is future by Ghosh et al.[21]. A back spread network is created which contains of a variable number of contribution nodes, ranging from 8 to 83, a solitary hidden layer with 125 bulges and one output node representative positive or negative for the assumed input. The input dataset predictable is a single cord of data, in the case of this paper the contribution data to a printing program. assumed the similarities in input data. Like preceding systems, the neural network has to be skilled prior to usage. Experiments are performed in binary different situations: ->For the black-box trials, the writers use only data passed to the package, without having Admission to the programs basis or state. -> For the white-box trials, in addition to data used in the black-box trials, they use internal program state data, which is lone available when having admission to the package source-code. |
E. Flow based intrusion detection
|
All the methods taken so far rely on the obtainability of detailed information confidential a single request or network packet. Circumstances with limited quantities of information in a request or where greatest of the traffic is encrypted will not deliver the data these procedures require. Sperotto [22] emphases on network intrusion discovery as opposed to web-based interruption detection, by looking at SSH and DNS data. Since SSH traffic is encoded, it is not possible as anspectator to detect anomalous conduct by looking at the payload. The experiential packets within a time edge are grouped together founded on properties they strength have in common, such as IP speeches, ports and protocol to form a movement. These flows have sure properties of their own, irrespective of the payload contents of separate packets, including movements per second, packets per additional, bytes per second and amount of packets in a flow. In this circumstance, the flows per second capacities are used to classify movements as benign or malicious. A perfect consisting of two states is built based on Markov Manacles. The two states indicate whichever activity, where SSH circulation was observer, or idleness. The dataset consists of actual traffic collected after the University of Twenty network. Only kind traffic is used to train the perfect. Based on this skilled model, threshold values can be allocated to traffic flows. Organization of the flows is done founded on these values, where movements exceeding a certain verge are marked as malicious. After exercise the model, two synthetic and two unique data sets are used for testing. The original data is network circulation captured from the University of Twenty network. Every of these data sets covers both hateful and normal traffic; the hateful data is manually branded for the datasets covering real network traffic. The consequences varied between the artificial and original data sets, where the consequences were significantly better for the artificial data sets. As before mentioned, there is always a skill of between a good discovery rate and a low false positive rate. |
CONCLUSION
|
This paper has obtainable idea about the arithmetical anomaly recognition of network circulation. Here paper studied aarithmetical approach to analysis the delivery of network traffic to know the normal network traffic conduct. This Paper has also deliberated flooding attacks. Most of the deluge attacks reviewed in this education is the new type of flood bouts which are more secretive yet cause more plain impacts of denial of facility, such as those attacks branded under the low-rate DoSbouts. This paper also discussed a technique to recognize irregularities in network traffic, based on a α-stable perfect and statistical theory testing. |
References
|
- http://www.cert.or
- M. Li. An approach to dependably identifying signs of DDOS deluge attacks based on LRD traffic pattern credit. Computers & Security, 23(7): 549-558, 2004.
- C.S. Sastry, S. Rawat and A.K. Pujari. Network circulation analysis using singular worth decomposition and multiscale alters. InformationSciences, 177(23): 5275-5291, 2007.
- M.F. Rohani, M.A. Maarof and A. Selamat.IncessantLoSS detection using iterative window founded on SOSS model and MLS method. InProceedings of the International Session on Computer and Communication Engineering, Kuala Lumpur, Malaysia, May 2000
- H.Hajji.Arithmetical analysis of network traffic for adaptive responsibilities detection. IEEE Transactions on Neural Networks, 16(5):1053–1063, September 2005.
- J. D. Brutlag. Abnormal behavior detection in period series for network monitoring. In LISA ’00: Minutes of the 14th USENIX conference onSystem management, pages 139–146, Berkeley, CA, USA, 2000.
- D. Rincón and S. Sallent. On-line division of non-stationary fractal network traffic with wavelet alters and Log-likelihood-based figures. LNCS, 3375: 110-123, 2005
- C. Douligeris and A. Mitrokotsa.DDoSbouts and defense mechanisms: organization and stateof- the-art. Computer Networks, 44(5): 643-666, 2004.
- P. García-Teodoro, J. Díaz-Verdejo and G. Maciá- Fernández. Anomaly-based network interruption detection: techniques, schemes andchallenges. Computers & Security, 28(1-2): 18-28, 2009.
- V. A. SIRIS and F. PAPAGALOU.Application of anomaly detection algorithms for detecting syn flooding attacks. In Proceedings of IEEEGlobal Telecommunications Conference (GLOBECOM ’04), volume 4, pages 2050–2054, Dallas, USA, 2004
|