Keywords
|
Data hiding, Steganography, LSB, DCT, Polynomial, Quality metrics. |
|
|
INTRODUCTION
|
The word steganography is derived from Greek words Steganos and graphia. Steganos means covered and graphia means writing. Thus steganography means covered writing which is an art of covert communication. The word steganography is invented by the Trithemium who done an explicit work on cryptography [1]. Steganography have been under practice from the ancient period. In the history Herodotus discuss several stories such as slave and the shaved head, that gives the evidence for the presence of the secret communication [1, 2, 3]. Later in the 15th century Aleneas proposed different steganography methods including information hiding in the earrings of women, message by pigeons etc [10, 1]. |
According to Abbas chedda, Italian mathematician Jerome cardem reinvents the Chinese ancient paper masking method. In 19th and 20th century, Nazis invented several methods during Second World War such as microdots, invisible ink, and null cipher. In 1945 Morse code was concealed in a drawing [2,4,5]. In the digital era, steganography plays significant role in many applications where secret communication is necessary. For example, military and intelligence agencies have to pass the information in a secured manner to the recipients in order to restrict the attacks by the enemies. Similarly in law enforcement, counter intelligence agencies, banking, business and trading etc. |
Basically steganography is a stream of data hiding. Data hiding is a broad researching area where data is embedded secretly in another file .The purpose is for either authentication or communication. But steganography is used for secured communication especially. Broad classification of data hiding and steganography is given below Figure 1 [1,7, 8]. There are four major challenges in the field of steganography [6, 7, 8].First one is to enhance the security for the communication. Secondly, quality of the stego image i.e., indistinguishable form of a stegoimage to maintain imperceptibility to the malicious user. Thirdly, improvement in the pay load capacity and fourth one is to improve the robustness against the attacks by the unauthorized user i.e., ability to withstand for modifications. |
In this paper, polynomial based image steganography using optimal LSB Pixel Adjustment method is implemented and compressions with respective to the LSB[11] and DCT[8, 6] image steganography on jpeg and bmp image formats is present. The performance analysis of these methods is done by measuring the objective and subjective quality metrics such as PSNR, NCCE, UIQI, SSIM[13]. |
II. METHODOLOGY
|
This paper presents the methodologies of LSB insertion method, DCT Steganography, POLPA Steganography briefly in below context. In the first LSB insertion based image steganography, secret information is embedding in the least significant bit of the each pixel in the cover image. These changes may not reflect the changes in the visualization of the cover image much but effects the statistical properties of it. |
Let us consider an 8-bit color image in which each pixel is represented with 24-bits. Since each pixel consists of three Components Red, Green, Blue. So, we can store 3 bits in each pixel. For example, the letter A can be hidden in three pixels. The original raster data for 3 pixels (9 bytes) may be |
(00100111 11101001 11001000) |
(00100111 11001000 11101001) |
(11001000 00100111 11101001) |
The binary value for A is 10000011. Inserting the binary value for A in the three pixels would result in |
(00100111 11101000 11001000) |
(00100110 11001000 11101000) |
(11001000 00100111 11101001) |
The underlined bits are the only three actually changed in the 8 bytes used. On average, LSB requires that only half the bits in an image be changed. You can hide data in the least and second least significant bits and still the human eye would not be able to discern it. The steps illustrate the embedding process of the message in the LSB’s of the cover image. |
Algorithm: |
Inputs : Secret message, cover image. |
Inputs : Secret message, cover image. |
Start |
Select the information to be hiding. |
Encode the secret information into binary form. |
Choose the suitable cover image. |
Encode the cover image into binary form. |
Check the size of the message and the cover image. |
If cover image is larger then |
Embed each bit of the secret information into LSB |
bit of each pixel in the cover image in an a predefined pattern. |
End |
The resultant image is called stegoimage and it is innocuous. |
End. |
The second method is DCT based image steganography. In this, embedding of the secret information is done in the frequency domain. DCT transform separates the cover image into spectral bands with respect to the low, high and moderate frequency components. As low frequency components of the cover image contains most of the image details apart from high and moderate frequency components, the secret information will be embedded in the low frequency components. Initially the DCT coefficients of the cover image are calculated based on the transformation synthesis equation. Then low frequency coefficients of the cover image are separated and embed the secret information. After that stego image is obtained by the transformation analysis equation. The complete block diagram of the DCT steganography is given in the figure 2 and step by step algorithm is below. |
Algorithm: |
Inputs : Secret message, cover image. |
Outputs: stegoimage. |
Start |
Select the information to be hiding. |
Encode the secret information into binary form. |
Choose the suitable cover image. |
Apply DCT transform to find coefficients of the cover image |
Select a set of low frequency DCT coefficients |
Encode the Selected coefficients into binary form. |
Check the size of the message and the selected DCT Coefficients. |
If no. of coefficients are larger then |
Embed each bit of the secret information into the lsb |
bit of each DCT coefficient of the cover image. |
End |
Apply Inverse DCT Transform to ob the spatial domain |
The resultant image is called stegoimage and it is innocuous. |
End. |
III. PROPOSED METHOD
|
The draw backs that are observed in the previous method such as statistical parameters of the cover image vary largely which degrades the quality of the image. This leads to identify the presence of the secret information perceptually to the malicious users. Even thought the extraction of the secret information is difficult, it loses significance of the steganography. Thus, we proposed a new method Polynomial based Optimal LSB Pixel Adjustment image steganography[7] that minimize the distortion in the stego image as well as the deviations in the statistical parameters, it enhance the quality stegoimage and improves the payload capacity of the secret information. The complete process of the proposed method will be represented in the following schematic Figure 3. |
Algorithm: |
Inputs : Secret message, cover image. |
Outputs: stegoimage. |
Start |
Select the information to be hiding. |
Encode the secret information into binary form. |
Choose the suitable cover image. |
Encode the cover image into binary form. |
Choose appropriate polynomial equation |
Find the set of pixels corresponding to the polynomial values. |
Check the size of the message and the no. of pixels. |
If set of pixels is larger then |
If pixel intensity >32 |
Embed 1-bit of the secret information into the lsb |
bit of each pixel in the cover image |
elseif 32<pixel>128 |
Embed 2-bit of the secret information into the lsb |
bit of each pixel in the cover image |
elseifpixel>128 |
Embed 3-bit of the secret information into the lsb |
bit of each pixel in the cover image |
End |
Adjust the pixels such that pixel difference with respect to the cover image is minimum |
End |
End |
The resultant image is called stegoimage and it is innocuous. |
IV. RESULTS AND DISCUSSIONS
|
Three steganography approaches using LSB, DCT and proposed method have been implemented in MATLAB bmp and jpeg images such as lenna.bmp, house.bmp and baby.jpeg, peppers.jpeg images. Figure 4 represents the text data chosen for hiding in an innocent cover image. In the Figure 5 stego images were compared with each other and with reference to the original or unmodified cover image. Figure 6&7 visualize the error between cover image and the stegoimage of the LSB, DCT, and POLPA steganography methods in histograms. Here histogram analysis is done for flexibility to observe the pixel difference from cover image to the modified cover image. Histogram analysis and comparison is done for between jpeg and bmp formats, since in images, discernable details of the picture vary with respect to the format. |
Stego Image quality analysis and comparison have been done by observing Table 1 & Table 2. UIQI and SSIM metrics give better understanding of features between stego and cover image in the human visual system for effective comparison than the objective metric such as PSNR and NCCE because subjective metric analysis concentrates on correlation, contrast and the brightness or the luminance. But objective metrics concentrates on the statistical properties of the image. From PSNR, NCCE,UIQI and SSIM observations it is clear that error between stego and cover image is significantly minimum for POLPA method with respect to the LSB and DCT method. For good quality image base line values are considered as 100 for PSNR and 1 for NCCE, UIQI, SSIM. |
V. CONCLUSIONS
|
In this paper, a new steganography approach POLPA along with LSB and DCT method was presented, implemented and analyzed. The proposed method hides the secret information based on polynomial values and adjusted in such a way that deviation in the quality of the stego image and the cover image is minimized with respect to the LSB and DCT approaches. Here steganography is implemented for jpeg and bmp image formats in order to test its efficiency. Quality measures were done by using PSNR, NCCE, UIQI and SSIM metrics. From the table and the fig. we conclude that the perceptual and statistical deviation is minimum in the POLPA than the LSB and DCT. |
|
Tables at a glance
|
 |
 |
Table 1 |
Table 2 |
|
Figures at a glance
|
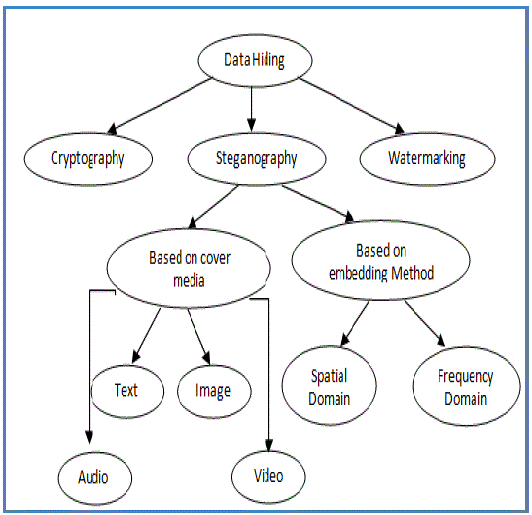 |
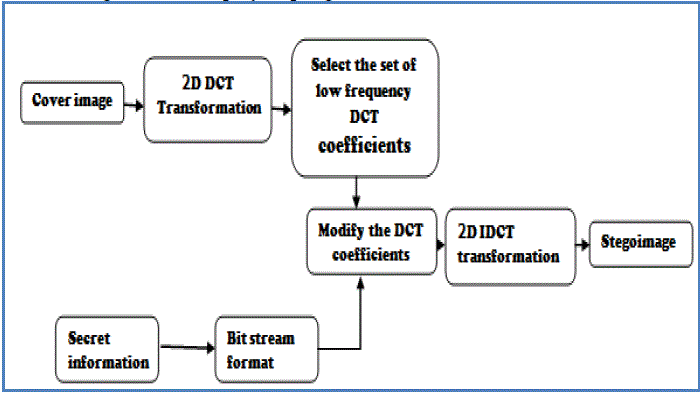 |
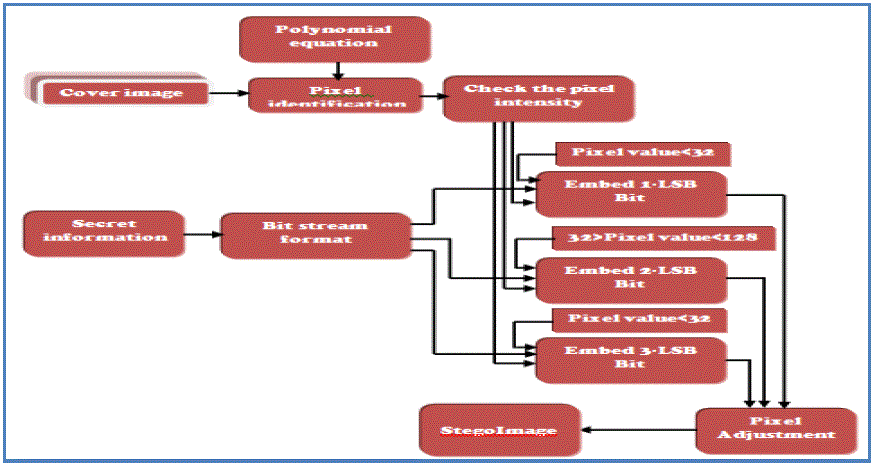 |
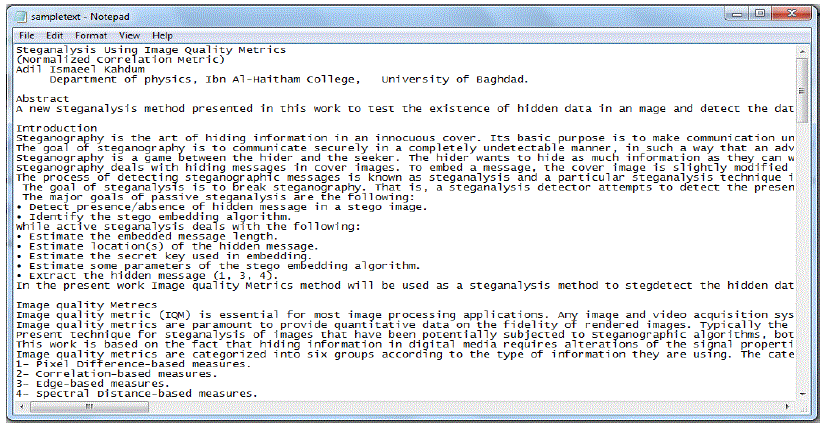 |
Figure 1 |
Figure 2 |
Figure 3 |
Figure 4 |
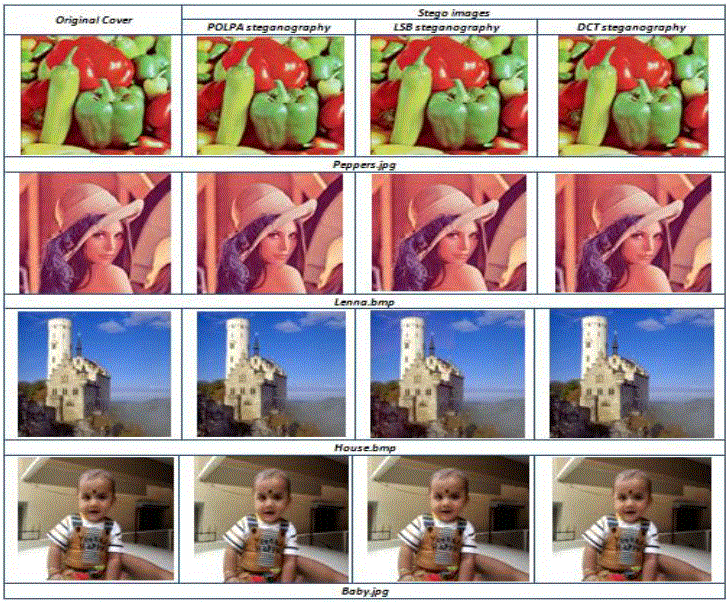 |
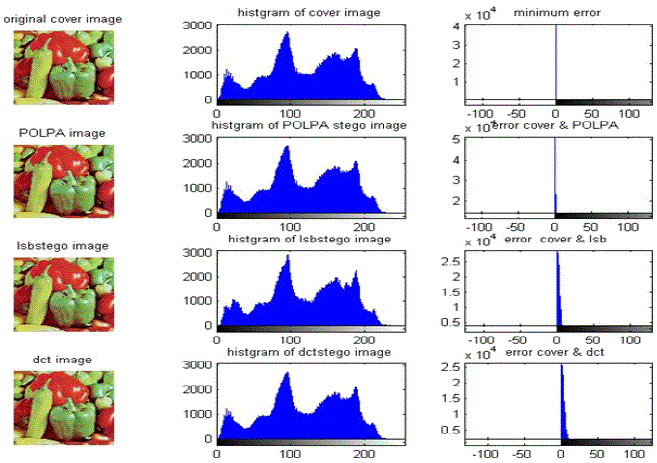 |
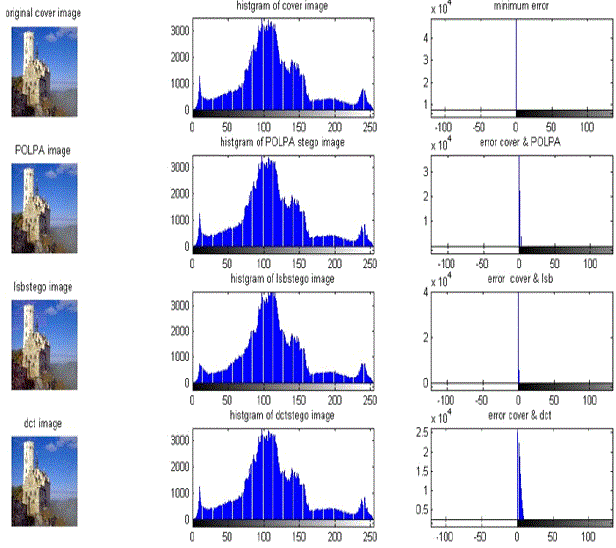 |
Figure 5 |
Figure 6 |
Figure 7 |
|
|
References
|
- Ingemar J. Cox, Matthew L. Miller, Jeffrey A. Bloom, Jessica Fridrich, Ton Kalker, “Digital Watermarking and Steganography”, SecondEdition, Morgan Kaufmann Publishers, ISBN 978-0-12-372585-1, 2008.
- James C. Judge, “Steganography: Past, Present, Future”, Information Security Reading Room,SANS Institute,2001.
- Nielsprovos, peter, Honeyman, “Hide and Seek: An Introduction to Steganography”, IEEE Computer Society, May/June,2003.
- N.F. Johnson, S. Jajodia, “Exploring steganography: seeing the unseen”, IEEE Computer 31 (2), 26–34, 1998.
- D. Kahn, “The codebreakers: the comprehensive history of secret communication from ancient times to the Internet”, Scribner, December 5,1996
- TVS Gowtham Prasad, Dr. S Varadarajan, r.S.A.KJilani , Dr. G N Kodandaramaiah, “Steganography Using Discrete Wavelet Transform”,IJART, Vol.2 Issue 2, ISSN NO: 6602 3127, 2012,.
- TVS Gowtham Prasad, Dr. S Varadarajan, Dr. S.A.K Jilani, Dr. G N Kodandaramaiah, “Image Steganography Based On Optimal LSB PixelAdjustment Method”, International Journal of Computers & Technology, Volume 5, No. 1, ISSN 2277-3061, May -June, 2013,.
- Ying Wang and Pierre Moulin, “Steganalysis of block-DCT Image Steganography”, University of Illinois at Urbana-Champaign, CCR 00-81268and CCR 02-08809.
- R.Amirtharajan, R. Akila, P.Deepikachowdavarapu, “A Comparative Analysis of Image Steganography”, International Journal of ComputerApplications (0975 – 8887)Volume 2 – No.3, May 2010.
- E. H. Wilkins, “ A History of Italian Literature”, Oxford University Press, London, 1954.
- Chi-Kwong Chan and L. M. Cheng, "Hiding Data in Images by Simple LSB Substitution", Pattern Recognition, vol. 37, no. 3, pp. 469 – 474, March 2004.
- Abbas Cheddad, JoanCondell, KevinCurran, Paul McKevitt , “Digital image steganography: Survey and analysis of current methods”, Signal Processing 90, 727–752, 2010.
- Yusra A. Y. Al-Najjar, Dr. Der Chen Soong, “Comparison of Image Quality Assessment: PSNR, HVS, SSIM, UIQI”, International Journal ofScientific & Engineering Research, Volume 3, Issue 8, ISSN 2229-5518, August-2012,.
|