Keywords
|
Non-sub sampled contour transform, Image decomposition, Medical Image fusion, Phase congruency, Fuzzy logic. |
INTRODUCTION
|
Medical image has different types of imaging techniques such as X-ray, magnetic resonance imaging (MRI), magnetic resonance angiography (MRA), computed tomography (CT), etc., these techniques are attracted increasing attention due to its critical role in medical diagnosis. However, provide limited information where some information is common, and some are unique. |
Clinical image analysis exploits the numerical representation of digital images to develop image processing techniques that facilitate computer-aided interpretation of medical images. The classical representations of medical images are printed on radiological film and visualized using a light box, interpretation necessarily subjective and qualitative [1]. However, most modern medical image acquisition systems generate digital images that can be processed by a computer and transferred over computer networks. Digital imaging allows extracting objective, quantitative parameters from the images by image analysis. Typical applications include for instance volume try of organs or lesions using MRI or CT, using MRI morpheme try of the brain, CT images are anatomical information from MRI with functional information from PET, or radiotherapy the planning of therapeutic interventions such as surgery. |
1.1 Multimodel Medical Image |
Multimodal medical images provide complementary information like the structural image in addition higher spatial resolution provides more anatomy information while the functional image contains functional information of tissues. The medical images are taken from different sensors at different time and at different view point .Before the images to be fused should be properly aligned and should have equal size which can be achieved from image registration techniques [4]. There are two categories in fusion techniques, one is the directly select pixels, blocks or regions from clear parts which in the spatial domain to compose fused images, another category is combing the coefficients in multi scale transform domain [6]. The main goal of image fusion is to provide a single fused image, which provides more accurate and reliable information than any individual source image and in which more features may be distinguishable [7]. |
LITERATURE SURVEY
|
A. Fusion Rules |
Image acquisition technology and the finally improvement of radiological image quality have led to an increasing clinical requirement and physician’s demand for quantitative image interpretation in routine practice, in image analysis imposing new and more challenging requirements[1] . The goal of image fusion is to extract information from input images in that the fused image provides better information for machine or human perception as compared to any of the input images [2]. Image fusion has been used extensively in various areas of image processing such as nondestructive evaluation, remote sensing, biomedical imaging, etc. |
A fusion rule is the processing that determines the formation of the fused source images, and it normally consists of four key components, i.e., coefficient grouping, coefficient combination, activity-level measurement, and consistency verification. The coefficient grouping schemes can be roughly divided into three categories, no grouping (NG), single-scale grouping (SG), and multiscale grouping (MG).The fusion decision for every set of corresponding detail coefficients at the current scale is made based on the sum of their activity levels and their corresponding coefficients at a higher scale [7]. Combination scheme for the choose-max (CM) strategy, i.e., selecting the coefficient with the highest activity level at each location from the MSR (Multiscale resolution)s of the source images as the coefficient at that location in the MSR of the fused image . Activity Level Measurement, It reflects the salience of each coefficient in multiscale resolution. Consistency Verification The consistency verification schemes ensure neighboring coefficients are fused in a similar manner. |
B. Multiscale Decomposition |
The wavelet transform (WT) and pyramid transform (PT) are the two multiscale decomposition schemes that are most commonly used in image fusion. A standard WT scheme is the discrete WT (DWT) which decomposes a signal into an MSR using scaling (low-pass filtering) and wavelet (high-pass filtering) functions. Although theoretically the decomposition of an image can be performed iteratively at low-resolution levels, which impairs the fusion quality. |
C. Phase Congruency |
Phase congruency is a measure of feature perception in the images which provides a illumination and contrast invariant feature extraction method. This approach is based on the Local Energy Model, which postulates that significant features can be found at points in an image where the Fourier components are maximally in phase. |
The main properties, which acted as the motivation to use phase congruency for multimodal fusion, are as follows. |
 |
The phase congruency feature is invariant to illumination and contrast changes. The capturing environment of different modalities varies and resulted in the change of illumination and contrast. Phase Congruency provides the improved localization of the image features, which lead to efficient fusion. |
PROPOSED IMAGE FUSION FRAME WORK
|
3.1 Image fusion |
Frequency bands obtained by the frame let transform are the approximate version of source images and usually include average gray and texture information. The simplest way is to use the conventional averaging method to produce the composite bands. However, it cannot give fused approximation of high quality for image because it leads to the reduced contrast in the fused images. |
3.2 Proposed Fusion Framework |
The proposed fusion framework will be discussed in detail. Considering, two perfectly registered source images A and B. The fusion approach consists of the following steps: |
1. Perform l -level NSCT on the source images to obtain one low-frequency and a series of high-frequency subimages at each level and direction, i.e., |
 |
 |
 |
RESULT AND DISCUSSION
|
Some general requirements for fusion algorithm are: (1) it should be able to extract complimentary features from input images, (2) it must not introduce artifacts or inconsistencies according to Human Visual System and (3) it should be robust and reliable. Generally, these can be evaluated subjectively or objectively. The former relies on human visual characteristics and the specialized knowledge of the observer, hence vague, time-consuming and poor-repeatable but are typically accurate if performed correctly. The other one is relatively formal and easily realized by the computer algorithms, which generally evaluate the similarity between the fused and source images. However, selecting a proper consistent criterion with the subjective assessment of the image quality is rigorous. Therefore, first an evaluation index system is established to evaluate the proposed fusion algorithm. These indices are determined according to the statistical parameters. |
The mean and variance of the images are calculated and the coefficients are selected using maximal variance scheme. Mean and variance can be calculated using following equation. |
 |
 |
 |
Where MxN is the size of the window , m(x,y) and σ(x,y) are the mean and variance . After selection of maximum values the fused image is resulted by taking the inverse wavelet transform of the detailed and approximate component of the fused image. |
The fig.4 described by the CT input images are (a) (d) (g) and (j), MRI input images are (b), (e) ,(h) and (k). Finally the Fused image gets the two image information in single output image. In this fusion process the more data and information are compared and fused in one outputs. CT image computed tomography (CT) can provide dense structures like bones and implants with less distortion, but it cannot detect physiological changes describe the bone structure of the brain, MRI image describe the brain blood flow activity and soft tissues. After getting fused image these two image information are shows one image. |
4.1Fusion of Noisy Multifocus Images |
To evaluate the performance of the proposed method in a noisy environment, the input multifocus images have been additionally corrupted with Gaussian noise, with standard deviation δ = 0.1, respectively. In the following experiment,since reference (everywhere-in-focus) image of the scene under analysis is not available; performance comparison of the proposed method cannot be made using mean square error (MSE). Therefore, image fusion performance evaluation measures to be employed. For comparison, besides visual observation, objective criteria on MI and QAB/F are used to evaluate how much information and edge information of the source clean images contained in the fused images. However, the objective criteria on MI and QAB/F cannot evaluate the performance of these fusion methods in terms of the input/output noise transmission. |
For further comparison, the improvement in terms of Peak Signal to Noise Ratio (PSNR), proposed by Artur Lozais is adopted to measure the noise change between the fused image and source noisy images. Let σ2 n,f denotes the noise variance in the fused output, the improvement in terms of Peak Signal to Noise Ratio (PSNR) as: |
 |
Mutual information (MI) is a quantitative measure of the mutual dependence of two variables. Mathematically, MI between two discrete random variables U and V is defined as |
 |
Where p (u, v) is the joint probability distribution function of U and V whereas p(u) are the marginal probability distribution function of U and V respectively. Based on the above definition, the quality of the fused image with respect to input images A and B can be expressed as |
 |
Where H(A), H(B) and H(F) is the marginal entropy of images A, B and F respectively. |
Edge Based Similarity Measure: The edge based similarity measure gives the similarity between the edges transferred in the fusion process. |
 |
Where A,B and F represent the input and fused images respectively. |
4.2 Analysis of fusion |
To verify the effectiveness of image fusion schemes explained some evaluation criteria is needed. Cross Entropy (CE):It measures the difference between the source image and fused image. Small value indicates better results .It is mathematically defined as : |
 |
Where qi is the gray level distribution of the fused image. Cross entropy due two images with respect to the fused image is determined. |
The Result of each input image Mean square value (MSE), Peak signal to noise ratio (PSNR) and Entropy pixel values is high after fusion process the entropy value is reduced and then PSNR value is increased. CT and MRI image each pixel value as shown in the above table. So the result of image quality is more and storage memory is reduced. |
CONCLUSION AND FUTURE ENHANCEMENT
|
A novel image fusion framework is proposed for multi-modal medical images, which is based on non-sub sampled contour let transform. For fusion, two different rules are used by which more information can be preserved in the fused image with improved quality. The low frequency bands are fused by considering phase congruency is adopted as the fusion measurement for high-frequency bands. In our experiment, two groups of CT/MRI images are fused using conventional fusion algorithms and the proposed framework. The visual and statistical comparisons demonstrate that the proposed algorithm can enhance the details of the fused image, and can improve the visual effect with much less information distortion than its competitors. These statistical assessment findings agree with the visual assessment. |
Future enhancement of multimodal medical image fusion is the combination of Wavelet transform and curve let transform namely wave atom transform for best fusion and contain more information in single image. |
Tables at a glance
|
 |
Table 1 |
|
Figures at a glance
|
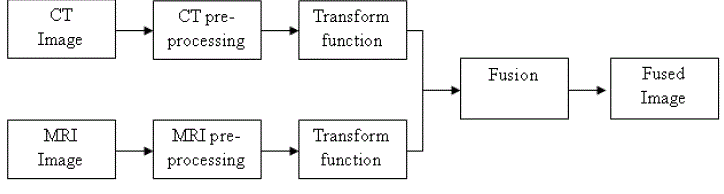 |
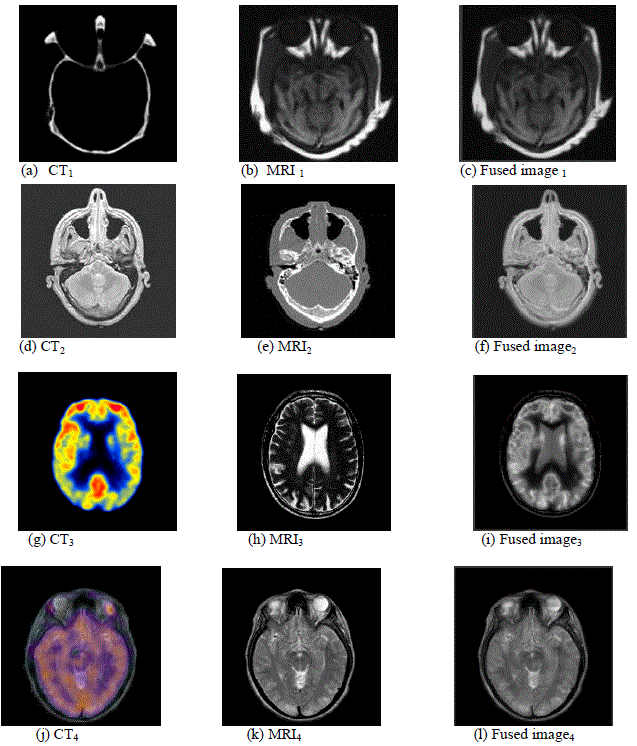 |
Figure 1 |
Figure 2 |
|
|
References
|
- Maes .F, Vandermeulen. D. and Suetens P. “Medical image registration using mutual information” , member, IEEE, vol. 91, no. 10, pp. 1699–1721,2003.
- Mrityunjay Kumar, and SaratDass, “A Total Variation-Based Algorithm for Pixel-Level Image Fusion” Member, IEEE, Image Processing, VOL.18, NO.9, pp. 2137–2143, 2009.
- MadhusmitaSahoo, Asst. Professor,” Biomedical Image Fusion &Segmentation using GLCM” Trident Academy of Technology, Bhubaneswar,India. IJCA Special Issue on “2nd National Conference- Computing, Communication and Sensor Network” CCSN, pp. 1–6, 2011.
- Changtao He, Guiqun Cao, ”An Efficient Fusion Approach for Multispectral and Panchromatic Medical Imaging” Fangnian Lang School ofElectronic Engineering, University of Electronic Science and Technology of China, West China Hospital, Sichuan University, China., BiomedicalEngineering Research, Vol. 2 Iss.1, PP. 30-36, 2013.
- RuiShen, Irene Cheng, and AnupBasu,”Cross-Scale Coefficient Selection for Volumetric Medical Image Fusion”, Senior Member, IEEE,Biomedical Engineering, Vol. 60, NO. 4, pp.1069-1079, 2013.
- Shutao Li, Xudong Kang, and Jianwen Hu,” Image Fusion with Guided Filtering” Member, IEEE, Image processing, Vol. 22, NO. 7, pp.2864-2875,2013.
- Liane C. Ramac and Pramod K.,” Application of Image Fusion to Wireless Image Transmission” Varshney EECS Department, Syracuse University,121 Link Hall, Syracuse, NY 13244,pp.1-6.
- Huiping Zhu1and Bin Wu1,”Medical Image Fusion Based on Wavelet Multi-Scale Decomposition” PengRen College of Information Engineering,Southwest University of Science and Technology, Mianyang, China .Journal of Signal and Information Processing, Vol. 4, pp 218-221, 2013.
- Gaurabhatnagar and Q. M. Jonathan,” An Image Fusion Framework Based on Human Visual System in Framelet Domain” Department of Electricaland Computer Engineering University of Windsor, Windsor Ontario, ON, N9B 3P4, Canada ., International Journal of Wavelets, Multiresolution andInformation Processing Vol. 10, No. 1, 1250002,pp.1-31, 2012.
- Nemir Al-Azzawi and Wan Ahmed K. “Medical Image Fusion Schemes using Contourlet Transform and PCA Based” , Wan Abdullah BiomedicalElectronics group, UniversitiSains Malaysia Penang, Malaysia., pp.93-111,2011.
|