Keywords
|
Direct Torque Control, MRAS, and Induction motor. |
INTRODUCTION
|
Sensor less based Direct Torque Control of induction motor drive has grown significantly in recent years due to its reduced hardware complexity, cost effective, elimination of sensor cable, increased reliability and less maintenance requirements [1], [2].The conventional Direct Torque Control principle is to controls the torque and flux directly or independently by selecting proper inverter switching states[3] that includes the hysteresis band controller which contributes ease of implementation and simple structure are the main advantages. It has main drawbacks of higher torque ripple, flux ripple and speed pulsation [4]. To solve the above problems, Neuro-fuzzy control schemes is introduced in DTC. Neuro-Fuzzy control together with space vector modulator is proposed in this paper .In this topology, Neuro-fuzzy controller calculate the reference voltage required to drive the flux and torque within a period of time with the help of torque and flux errors and calculated voltage is synthesized using SVM[5],[6].To achieve better torque and smooth flux control in induction motor drives, various sensorless-based methods have been used for speed estimation and give a good performance in large speed range. The different methods are speed adaptive flux observer [7], [8], model reference adaptive system [9],[10], slip calculation method and extended kalman filter [11],[12].Among various machine model-based methods, Model Reference Adaptive System(MRAS) appeared to be the best solutions for speed sensorless drives[13],[14].This paper proposes the Model reference adaptive system which is used to estimate the rotor speed with the help of machine terminal voltages and currents. Fuzzy control is used as an adaptive mechanism instead of PI to obtain accurate speed. From various intelligent algorithms, fuzzy logic is the simplest, and it does not require any intensive mathematical analysis. MRAS observer is widely preferred due to its ease of implementation with high speed of adaptation for wide range of applications, although this approach leads to small variations in low speed region. To estimate the stability of closed loop speed, the Popov’s hyper stability criterion is used [5], [6]. The proposed control scheme for sensorless based DTC-SVM is elaborately discussed in this paper. |
Section 1 describes the mathematical model of Induction Motor drive. Section 2 describes the proposed sensorless drives and DTC-SVM. Section 4 shows the simulation results for DTC, Sensorless DTC and Sensorless based DTCSVM. Section 5 gives conclusion and future works. |
SENSORLESS BASED DTC-SVM
|
A. Machine Equations |
The behaviour of IM is analysed with the use of space phase quantities in a stationary reference frame. The voltage balance equations for the d-q axes are as follows. |
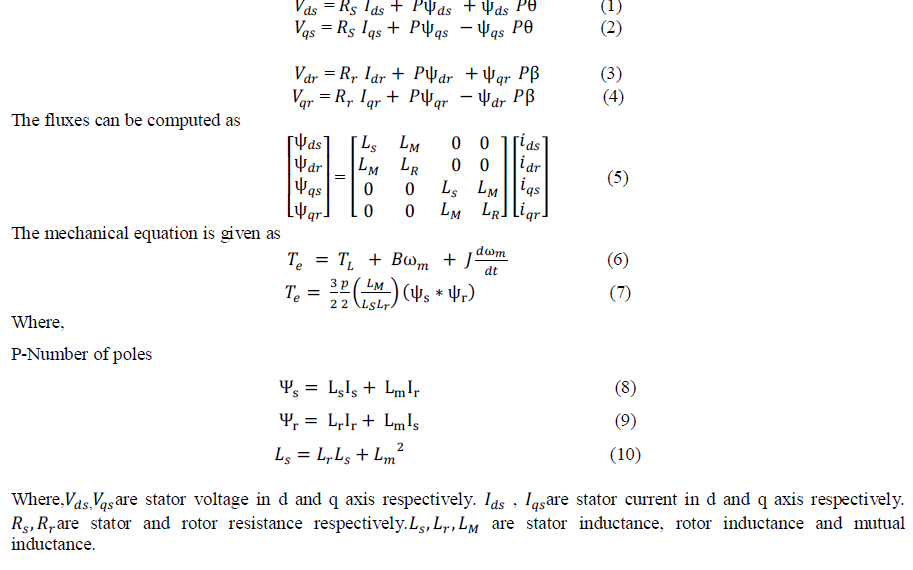 |
 |
B. Proposed Model Reference Adaptive System |
The proposed structure is a model-reference adaptive system MRAS, it consists of three steps: reference model, adaptive model and an adaptive method. Both models are referred to in the stationary reference frame. Fuzzy control is used as Adaptive mechanism instead of PI. The output of a reference model is compared with the output of an adjustable or adaptive model until the errors between the two models vanish to zero. The proposed sensorless drive is presented in Fig.1. |
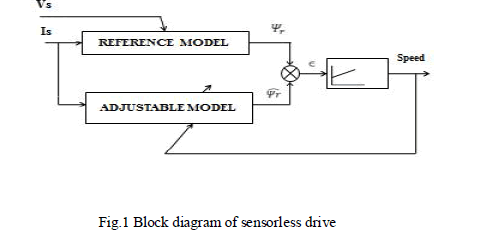 |
1) Reference Model: This model receives the machine stator side voltage and current signals and calculates the rotor flux vector signals. The stator and rotor flux linkages in the stator reference frame are defined as |
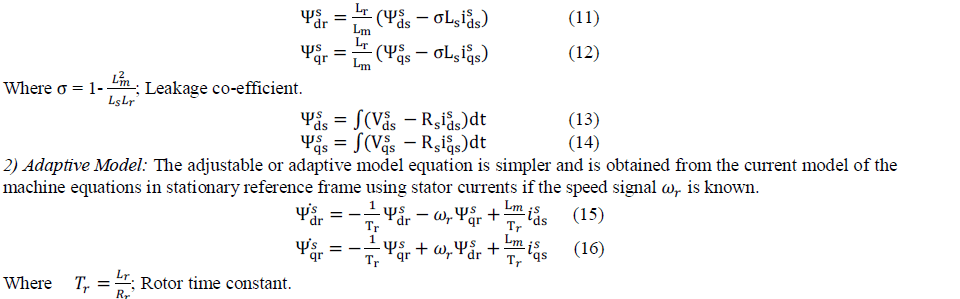 |
With the correct speed signal, fluxes calculated from reference model matches with the adaptive model. |
3) Adaptation Algorithm: An adaptation algorithm with fuzzy control can be used to tune the speed when the error = 0.Fuzzy logic control is the process of formulating the mapping from a given input to an output using fuzzy logic. It has some advantages such as the fact that it does not require an exact mathematical method. The rules for Fuzzy logic controller are shown in Table I. |
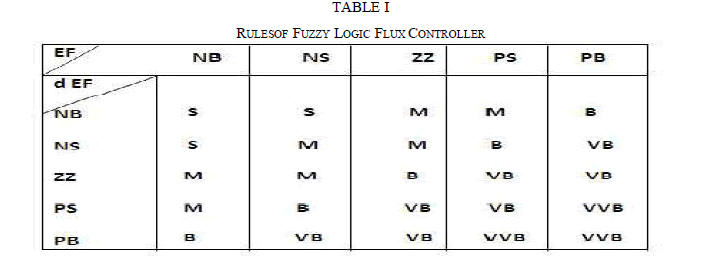 |
The torque reference is compared with the torque estimation and the error is the input to the fuzzy logic controller. Estimation accuracy is good if parameter variation is considered as constant. |
C. Direct Torque Control with SVM |
In Direct torque control, it is possible to control directly the stator flux and the torque by selecting the appropriate inverter state. To overcome the classical DTC drawbacks like increased torque ripple, SVM based DTC is used here. The block diagram of sensorless based DTC with SVM is shown in Fig 2. |
The proposed topology of the DTC-SVM comprises two Neuro-Fuzzy controllers for flux and torque. The controllers receive its inputs in the form of torque and stator flux errors and evaluate the reference voltage required to drive the flux and torque. This calculated voltage is then synthesized with the help of SVM. Neuro-Fuzzy acts on the magnitude of reference voltage whereas the angle is chosen from a table. |
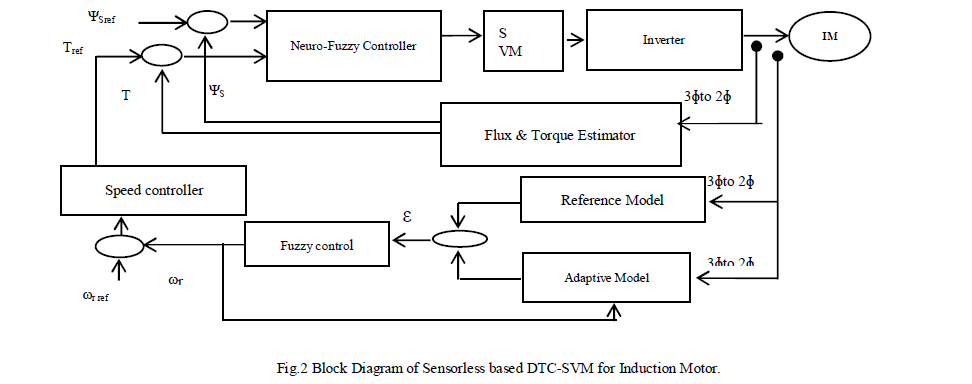 |
The SVM unit produces the inverter control signals. It receives the reference voltage in stator reference frame. SVPWM is a digital modulation technique which treats sinusoidal voltage as a constant vector rotating at constant frequency. This PWM technique approximates the reference voltage VREF by combination of the eight switching patterns (V0 to V7).Figure shows space vector representation. |
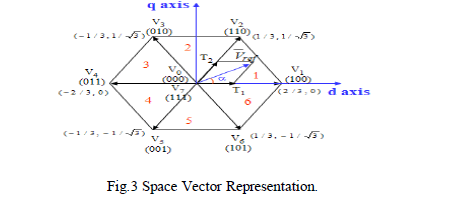 |
Steps involved in implementing the space vector PWM is given as |
 |
SIMULATION RESULTS
|
To validate the effectiveness of the MRAS based DTC-SVM methods, a two-level sensor less based DTC-SVM motor drive was developed in MATLAB environment and simulation results are presented here in Fig 6. The Model Reference Adaptive System based DTC drive is illustrated in Fig. 1. |
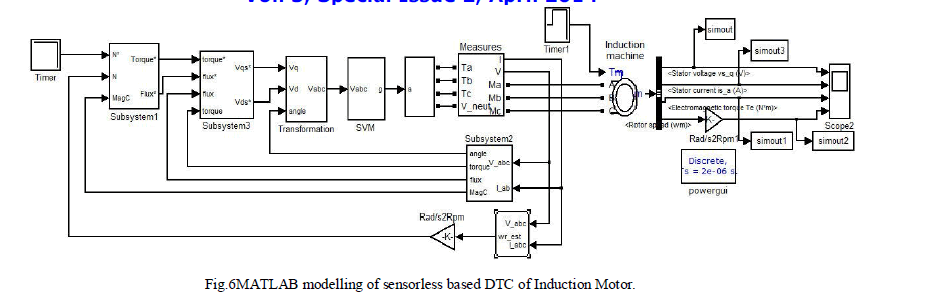 |
Sensorless MRAS technique is employed for closed loop speed estimation. A simulation work has been carried out on an induction motor with the specifications given in appendix. The proposed MRAS scheme is simulated in MATLAB/SIMULINK which is shown in Fig.7.Fig. 8 and Fig. 9 shows stator current for DTC of induction motor and sensorless based DTC of induction motor. |
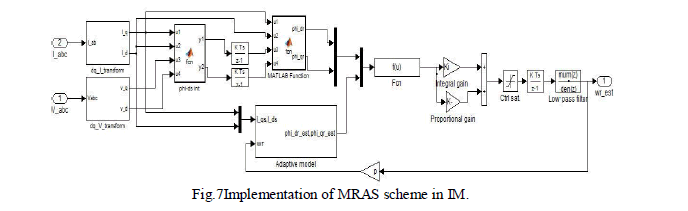 |
When load torque of 500Nm is applied at time t = 0.3s in classical DTC and sensorless based DTC, same magnitude of stator current is flow in induction motor. But current pulsation in sensorless based DTC is comparatively reduced. |
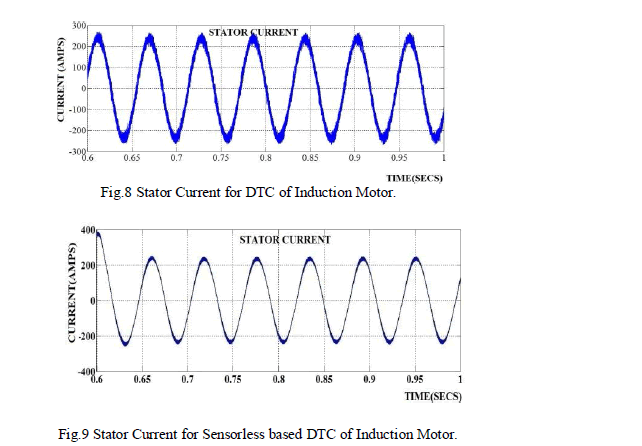 |
Stator flux of direct torque control and sensorless based direct torque control of induction motor is shown in Fig. 10 and Fig. 11.When compared with DTC, stator flux is more uniform in sensorless based DTC. |
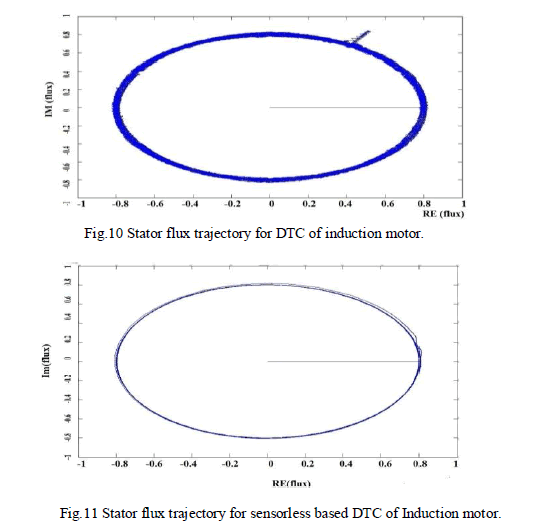 |
Electromagnetic torque response for DTC and sensorless based DTC for induction motor is shown in Fig.12 and Fig. 14.The Fig. 12 shows the electromagnetic torque for DTC of induction motor. Initially the motor run with a electromagnetic torque of 300 Nm. At t = 0.3 s, the load torque of 500 Nm is applied to the motor shaft while the motor speed is still ramping to its final value. This forces the electromagnetic torque to increase to the maximum value and then to stabilize around 500 Nm once the speed ramping is completed. Speed ramping is completed at t = 0.6s. |
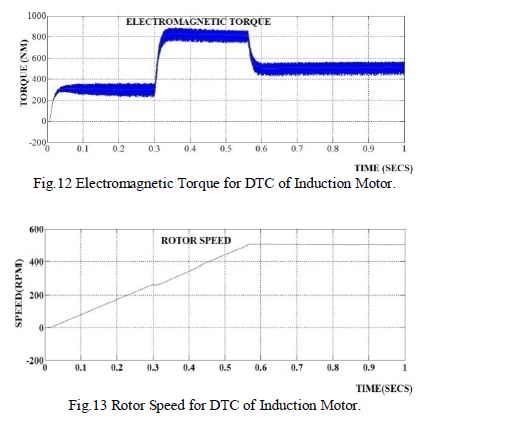 |
When load torque of 500 Nm is applied, output torque oscillates between maximum and minimum torque of 550 Nm and 430 Nm respectively and rotor speed ramping to 500 rpm and remain constant for remaining periods in both DTC and DTC-sensorless of induction motor which is presented in Fig. 13 and Fig. 14. |
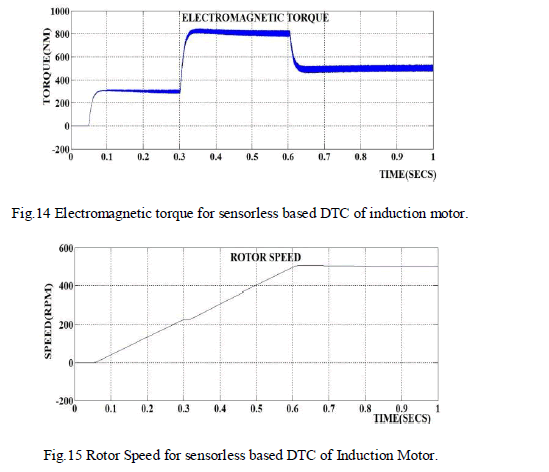 |
In sensorless based direct torque control when motor is starts with zero load torque at t=0s, it will oscillates and settles to its steady state with 300Nm initially. When load torque of 500 Nm is applied at 0.3 seconds applied, motor oscillates and then settles at steady state. In steady state the load torque oscillates between maximum and minimum value of 520 Nm to 460 Nm. |
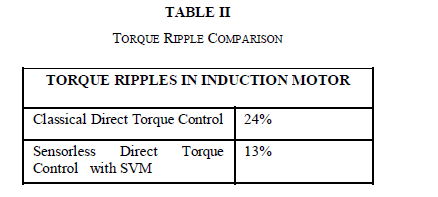 |
Comparison of torque ripple in DTC and DTC with sensorless is shown in Table II. Therefore from results in table it is inferred that proposed scheme has less torque ripple, smooth flux response and less current pulsation. |
CONCLUSION
|
In this paper, sensorless based direct torque control technology for induction machine has been proposed. Speed estimation is achieved using model reference adaptive system which eliminates the need speed sensor. Sensorless based DTC improve induction motor performance in terms of flux, torque and current response. Application of Neuro-Fuzzy in error minimization in MRAS will gives more accurate speed estimation. The simulation results verify that the proposed scheme improves the performance of induction motor. |
APPENDIX
|
Motor parameter used in the simulation: |
Induction Motor Detail |
460V, 149.2KW, 4 Poles, 1785 rpm |
Stator resistance 14.85e-3 ohm |
Stator inductance 0.3027 mH |
Moment of inertia 3.1 kg.m2 |
Friction coefficient 0.08 N.m.s/rad |
References
|
- DomenicoCasadei, Giovanni Serra, Angelo Tani, Luca Zarri, and Francesco Profumo,” Performance Analysis of a Speed-SensorlessInductionMotor Drive Based on a Constant-Switching-Frequency DTC Scheme” IEEE Transactions On Industry Applications, VOL. 39, NO. 2,pp. 476-484, March/April 2003.
- Haithem Abu-Rub, JaroslawGuzinski, ZbigniewKrzeminski, and Hamid A. Toliyat, “Speed Observer System for Advanced SensorlessControlof Induction Motor” IEEE Transactions On Energy Conversion, VOL. 18, NO. 2,pp.219-224 june 2003.
- Takahashi and T. Noguchi, “A newquick-response and high-efficiency control strategy of an induction motor,” IEEE Trans. Ind. Applicat.,vol.IA-22, pp. 820–827, Sept./Oct. 1986.
- D. Casadei, G. Grandi, G. Serra, and A. Tani, “Effects of flux and torque hysteresis band amplitude in direct torque control of inductionmachines,” in Proc. IECON’94, Bologna, Italy, Sept. 5–9, 1994, pp.299–304.
- D. Casadei, G. Sera, and A. Tani, “Stator flux vector control for high performance induction motor drives using space vector modulation,” inProc. OPTIM’96, 1996, pp. 1413–1422.
- P. Thoegersen and J. K. Pedersen, “Stator flux oriented asynchronous vector modulation for AC-drives,” in Proc. IEEE PESC’90, 1990, pp. 641–648.
- Paladugu, B. H. Chowdhury, “Sensorless control of inverter-fed induction motor drives”, Electric Power Systems Research 77 (2007) 619-629.
- M.Juili, K.Jarray, Y.Koubaa, M.Boussak, “Lenberger state observer for speed sensorless ISFOC induction motor drives”, Electric Power SystemsResearch 89 (2012) 139-147.
- T. Ravi kumar, Ch. Shankar Rao, Ravi Shankar “Model Reference Adaptive Technique For Sensorless Speed Control Of InductionMotor”International Journal Of Engineering And Computer Science ISSN:2319-7242 Volume 2 Issue 5 May, 2013 Page No. 1578-1583.
- M.K. Metwally “Sensorless speed control of 4-switch three phase inverter fed induction motor drives at very low and zero speed” AlexandriaEngineering Journal (2013).
- Salomón Chávez Velázquez, Rubén Alejosalomares, Alfredo Nava Segura “Speed Estimation for an Induction Motor Using the ExtendedKalman Filter”, Proceedings of the 14th International Conference on Electronics,Communications and Computers (CONIELECOMP’04) 0-7695-2074-X/04 $ 20.00 © 2004 IEEE.
- Americo Vicente Leite, RuiEstevesAraujo, , and DiamantinoFreitas “A New Approach for Speed Estimation in Induction Motor Drives Basedon a Reduced-Order Extended Kalman Filter” 0-7803-8304-4/04/$20.00 C02004.
- D. P. Marcetic, S. N. Vukasavic, “ Speed-Sensorless AC Drive With the Rotor Time Constant Parameter Update”,IEEE Trans on industrialelectronics, VOL. 54,NO. 5,October 2007.
- P. Jansen, R. Lorenz, and D. Novotny, “Observer-based direct field orientation: analysis and comparison of alternative methods,”IndustryApplications, IEEE Transactions on, vol. 30, no. 4, pp. 945–953, 1994.
- Y.D.Landau, Adaptive control- The Model Referencing Apprach, Marcel Dekker, 1979.
|